In 2012, Benoit Dageville and Thierry Cruanes, the integral members of a veteran group of Oracle data architects, wanted to identify better ways to store data than their on-premise data solutions. Meanwhile, Hadoop was a transformative product the industry had seen, and Cloudera came into momentum in 2014.
They came up with the idea of building a cloud-native data warehouse in 2012 riding the wave of cloud adoption. The first product was launched in 2014. Snowflakes came into existence.
By August 2022, it was grown to more than $50 billion in market cap. It became the stage for modernized data platforms.
Databricks followed soon after in 2013. It emerged from a university research project from the AMPLab project at the University of California, Berkley. Ali Ghodsi and co-researchers developed Apache Spark, a faster alternative to Hadoop. Then they worked to create the open source version of Spark, Databricks. Databricks was valued in a private venture round at $38 billion in August 2021.
In 2023 both the titans became the giants in the industry. Their products represent the massive shift towards changing ways to manage and analyze data.
Traditionally companies used an on-premise data warehouse to store the data and run the after-the-fact analysis with BI dashboards. The rise of the cloud, along with tools like Databricks and Snowflake, has enabled this growing pool of data to help companies identify insights, improve products and make informed decisions.
What is the usage of Snowflakes and Databricks?
To understand the contrast between Snowflake and Databricks, you must understand AI. The greatest misconception among executives is that AI can be easily used on data and derive insights overnight. Instead, building an effective AI model that delivers value for a business is a defined process.
This involves data gathering, cleaning, and preparation to build a model around that data. After multiple iterations, a model is ready to be operationalized.
Snowflakes and Databricks create value at different layers of this whole stack.
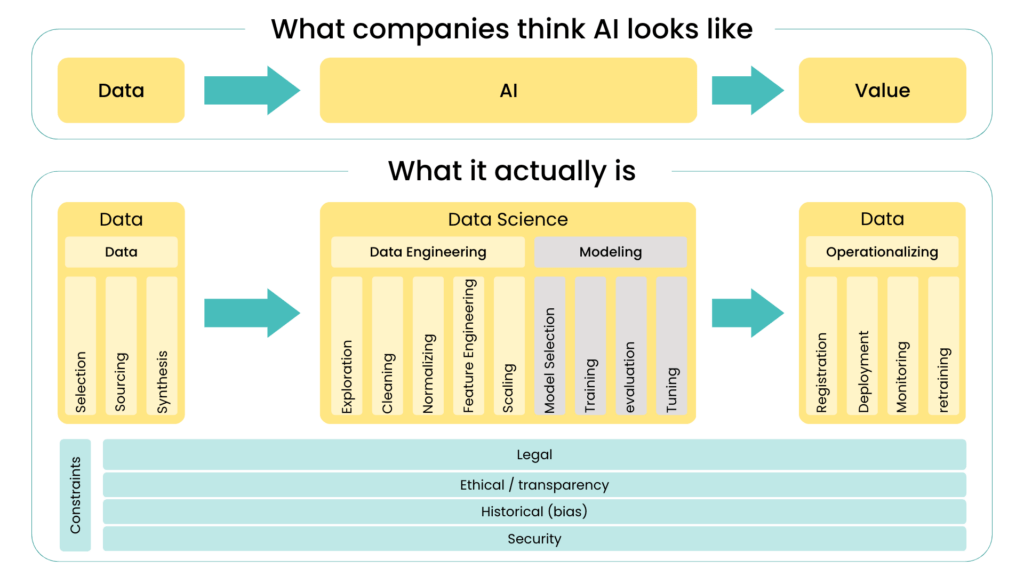
In the diagram above, Snowflake focuses on the left, from data storage to data engineering, including most of the components at the bottom, such as implementing security and legal policies on data.
On the other hand, Databricks historically has focussed more on the steps from data modeling to operationalizing data models.
Implementing analytics and big data for every organization starts with understanding “What happened?” versus “What will happen?”
Data warehouses store the data required to answer “What happened?” and Data lakes manage the data required for building models to answer “What will happen?”.
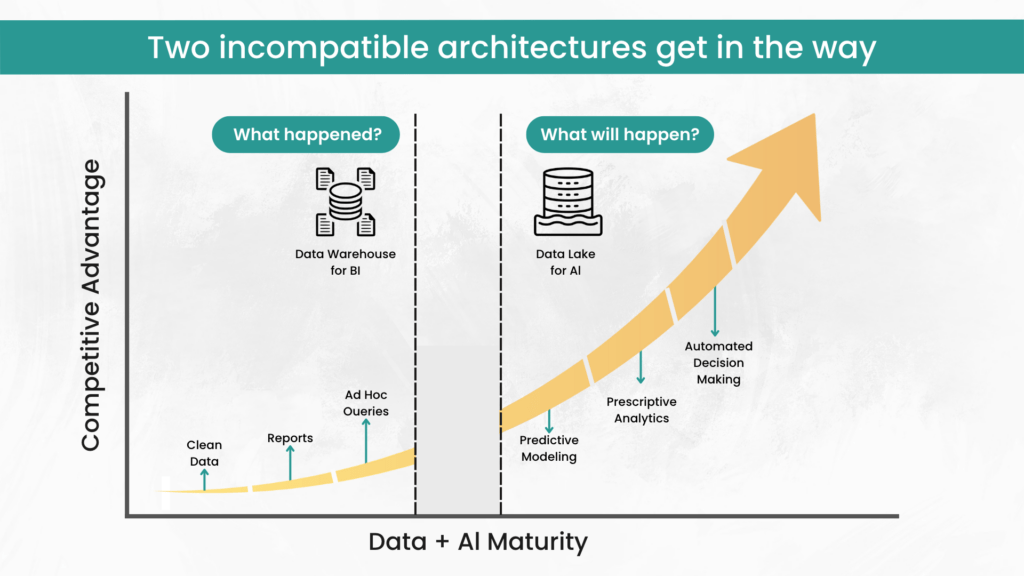
What is Snowflake?
It is a cloud data platform built on the foundation of a data warehouse. It enables customers to consolidate data into a single source of truth and drive meaningful business insights, build data-driven applications, and share data.
The key features include the following –
- Flexible scaling to handle any amount of data
- Nil management is required for hardware, software, or tuning
- In-built security features to protect your data
- Supports a variety of data sources and integration with BI tools
What is Databricks?
Databricks is similarly a cloud data platform built on the foundations of the data lake. The data lake is used for data storage, but its purpose is to enable data scientists to leverage machine learning applications to analyze the data. The platform is primarily geared toward data science and machine learning applications.
The key features include the following –
- Unified system for data users
- Collaboration tools to enable teams to work together on data projects
- Automated machine learning to simplify model building and deployment
- Integration with popular big data frameworks like Spark and Hadoop
Databricks and snowflakes have empowered thousands of companies to access, explore, share, and unlock the value of their data. Their platforms have leveraged the elasticity and performance of the cloud to solve data silos. Both platforms enable customers to unify and query data to support various use cases.
Two platforms with the same vision
Both companies are chasing the same vision of becoming the modern enterprise’s single “big data” platform.
- Cost savings and seamless data transfer without ETL
Continuous innovations in the space have reduced compute and storage costs. Snowflake’s most significant innovation since its inception was the launch of Unistore and hybrid tables. Customers can save costs by avoiding purchasing extra software such as Fivetran or DBT Labs.
- Multi-cloud benefits
The shift from on-premises to the cloud has been the most important catalyst for both companies. Databricks and Snowflakes benefit from being multi-cloud. This enables customers to adopt Amazon AWS, Microsoft Azure, and Google Cloud Platform. At their recent data+AI summit they claimed that over 80% of their enterprise customers use more than one cloud.
Different Approach
Data Science vs. Data Analytics
Databricks originated as a data lake built around open-source Spark for data science and ML use cases. Meanwhile, Snowflake is built as a cloud data warehouse that could be used for business intelligence analytics.
- Open vs. Closed Ecosystem
The significant difference between both them is the data source. Snowflake is widely considered a closed platform because the entire storage and compute platform is in a closed ecosystem. On the other hand, Databricks is open source. All their key product lines, from Delta lake to delta sharing, can be implemented for free. Customers can turn to Databricks enterprise offerings for more advanced features and support.
- Pre-Packaged vs. Configurable
Snowflake provides a packaged, off-the-shelf solution allowing companies to get basic analytics in minutes. Databricks offers much greater customization and configuration; customers have complete control over the setup.
- Comparing the Performance
Snowflake’s elastic scaling allows it to handle massive amounts of data without any performance loss. At the same time, Databricks’ optimized Spark engine makes it a powerful tool for data processing and machine learning.
The factors on which performance can be compared are –
1. Scalability–
Both excel in this area. Scaling up or down as per requirement is easier with Snowflake’s architecture, making it an ideal option for businesses that handle large amounts of data. Databricks can also handle massive amounts of data and provide real-time processing and analytics.
2. Architecture –
- Data Architecture – Snowflake is a cloud-based data warehousing platform that uses a multi-cluster shared data architecture. Data is stored in separate compute and storage layers, allowing separate scaling of compute and storage resources. The data is stored in proprietary columnar format for efficient querying and compression.
While Databricks unified data analytics platform uses a distributed computing architecture. It leverages Apache Spark as its processing engine and supports various data sources and file formats. The data is preserved in a spread files system like Hadoop or AWS S3 and processed using Spark’s in-memory computing capacities.
- Processing Architecture – Snowflake uses a shared-nothing processing framework. Each compute node has its CPU, memory, and storage and processes the data independently. This allows easy scaling by adding more compute nodes to the cluster.
On the other hand, Databricks uses a distributed processing architecture with clusters on worker nodes processing data in parallel. It uses Spark’s RDD (Resilient Distributed Datasets) abstraction to manage data processing and distribution across the cluster.
- Security Architecture – Snowflake’s security architecture is designed to keep customer data secure, including encryption at rest and in transit, network isolation, and user and role-based access control. It also includes data masking and secure views to protect sensitive data.
Databricks also provides robust security measures, including rest and transit encryption, network isolation and use, and role-based access control. It also consists of in-built integration with identity and access management (IAM) systems, that allow more fine-grained control over access to data and resources.
3. Costing –
Both of them offer a variety of pricing options. Snowflake’s pricing
is based on usage. The pricing models change as per the features and resources required.
4. USPs
Snowflake is known for its easy integration with SaaS applications. The cloud-native architecture and extensive set of APIs and connectors make it simple for users to connect to a wide range of SaaS tools and platforms such as Salesforce, AWS, Microsoft Azure, and Google Cloud Platform. Also, its flexible data-sharing capabilities enables seamless collaboration between different organizations, making it an ideal choice for SaaS companies that need to share data with their customers and partners securely.
Databricks introduced Delta Lake, an open-source storage layer that brings ACID transactions, versioning, and schema enforcement to data lakes. It enables users to build reliable and scalable data pipelines with improved performance. Databricks is built on Apache Spark, an open-source distributed data processing engine. It enables advanced data processing tasks such as ETL, machine learning, and graph processing.
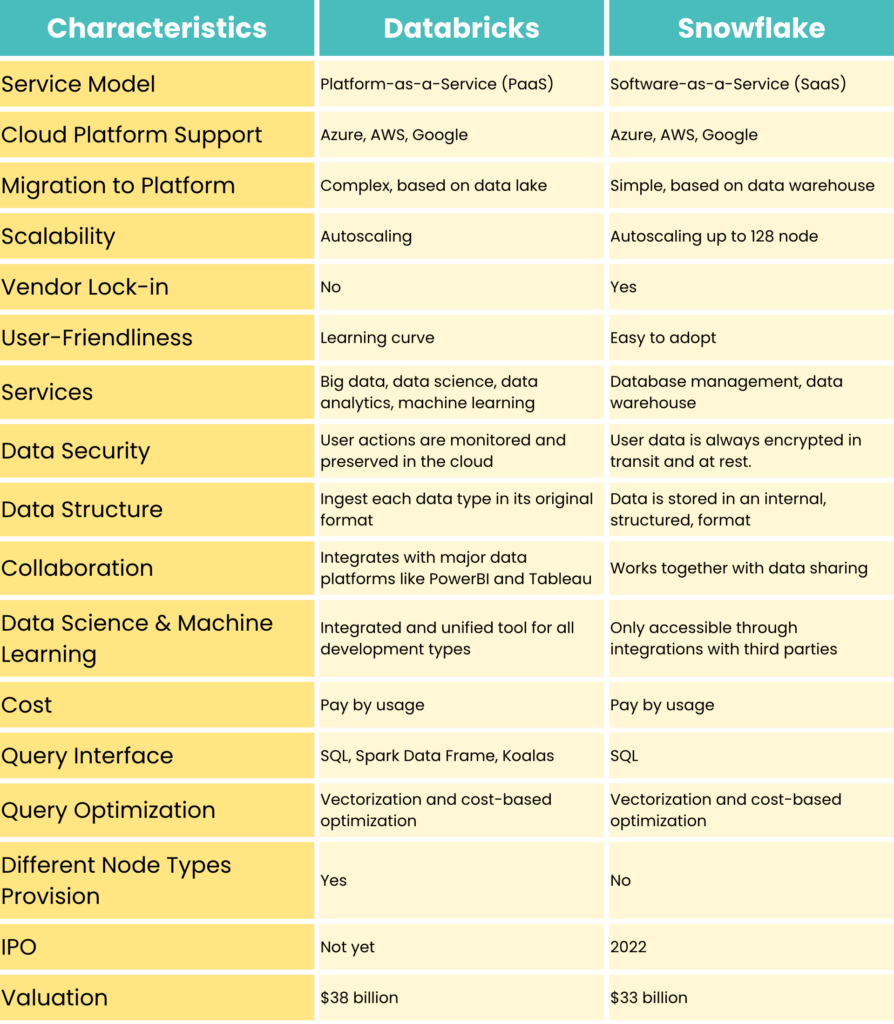
How 47Billion utilized Databricks for delivering a scalable Sales & Marketing Analytics solution for a senior living advisory firm?
A senior living advisory service in North America has a network of 17000 communities and home care providers. It operates a trusted online platform that connects families for senior care services. The organization helps hundreds of thousands of families make the right choice for their loved ones every year.
They required a scalable analytics and visualization solution to understand their sales and marketing activities and tactics.
47Billion worked collaboratively with their team to understand their challenges regarding the technology stack, efficient analytics process, and primary KPIs to be taken under consideration and helped build a scalable analytics and visualization solution.
Read the success story. Sales & Marketing Analytics for Senior Living Advisory Services Organization
When to choose Snowflake or Databricks?
You need to consider several factors while choosing the right platform. Here are some scenarios –
Cloud Data Warehousing – If your organization requires a scalable, cloud-based data warehousing solution that handles large volumes of data, Snowflakes may be a practical choice. Its multi-cluster shared data architecture allows for separate scaling of compute and storage resources.
Data Analytics and Machine Learning – Databricks would be a suitable option in this case. Its spread processing architecture using Apache Spark and support for various programming languages like Python, R, Scala, and Java make it a favorable choice.
Business Intelligence – Snowflake is a suitable choice if your organization needs to integrate with BI tools and support standard SQL. Its broad compatibility with BI tools like Tableau, PowerBI, and Excel and its compatibility with SQL makes it a popular choice for BI implementations.
Security and Compliance – Both provide robust security features and have compliance certifications such as SOC2, HIPPA, and GDPR. However, Snowflake’s security architecture is designed to keep customer data secure and has data masking, secure views, and network isolation.
Conclusion
What do you feel Snowflake or Databricks?
Both offer potent capabilities for managing and analyzing data. You need to choose the best platform for your business requirements. Whether you choose Snowflake or Databricks, you are sufficiently equipped to handle your data needs and gain valuable insights for better decision-making.
Building the universal data stack will require a platform that extends from simple data storage to complex machine learning models. Both companies are positioned to capitalize on the explosion of data. They have rapid product velocity and significantly higher ratings. The clash goes beyond simply building a business in a diverse market. Both are capable enough to become universal data platforms.